Colorization Chapter
The portrait experiment
This is a lower-resolution page, more convenient for an overview. Click on images to zoom.
All paintings below are, if I do not mistake, in the public domain and consequently free of rights.
Page map: training set / test set / results (to be updated regularily)
Training set (53 images)
These images are given as examples, in order to know what a color image looks like.
Test images (5 images)
The following images are converted into grey-scale values, and will be used later as test images.
Note that there can be issues concerning the true colors of a painting. For example compare :
Results
I modified the original training set, removing two images and down-sampling the biggest images, so that it fits the RAM during learning and prediction without requiring a program structure change; I updated the training set above accordingly.
The following result is based on all the training images above. Same parameters for the all test images.
Pixels on a 3x3 grid only were colored.
For each test image are displayed:
- image to be colored
- result
- color chosen, without grey level
- edge prediction (black = possible strong color variation, white = no possible color variation)
- most probable color at the pixel level
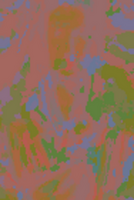
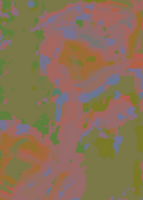
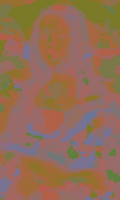
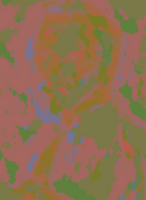
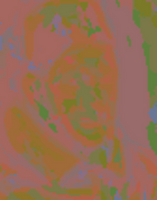
Notes :
- the edge (= color variation) prediction is quite good, much better than in previous experiments with very-small-sized training sets, and better than in the experiment with the 20-houses set. This indicates that the kernel parameters (number of nearest neighbors and standard deviation of the Gaussian) are correct.
- Locally, green is more often predicted than expected, even in the middle of faces.
- Blue artefacts disappear with the spatial regularization.
- One cannot reasonably expect parts other than the face to be colorized correctly, given the training set.
- Adding a new test image takes about half an hour (because of the search, for each pixel, for the 1000 nearest neighbors in the learning set); but a re-colorization with a different spatial coherency parameter takes only a few seconds.
- The spatial coherency parameter is the most important one, but, apparently, the one for smoothing the color probabilities in the color space, as well as the precise way color variation predictions are handle, are important too.
Now let us perform other tries, with different values for the parameter of color spatial coherency, all other parameters being kept constant (to the values used in the first experiment, not shown here).
Have a look at the summary page with all results of this set of experiments, practical to see the effects of the spatial coherency parameter (you can click on one experiment to access its personal web page).
Summary for a second set of experiments with more parameters varying. The experiment shown above is experiment 45.
Back to the colorization page.
Back to the main page